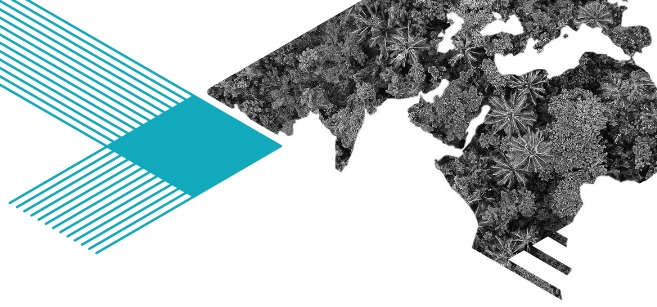
Imperial College London
Added-value content for members of the world-leading university’s business partnership scheme, promoting specialist consultancy offer.
Relevant if you need: white papers, research, reports, research, interviews, technical…
The brief:
To interview academics using AI across a range of disciplines, finding points of connection in their work and creating a clear story around the resulting consultancy offer to businesses and organisations involved in risk management.
The challenge:
Mastering distinct scientific and financial subject areas, and communicating a specialist offer in a clear, accessible and urgent story, without losing the specificity at the heart of the value proposition.
How it went:
“I can thoroughly recommend Phil. He's excellent at capturing technical material from a wide range of scientific fields and rendering it in a compelling and accessible story for a business audience. Crucially, he's also reliable and easy to work with.” David Silverman, Enterprise News and Editorial Content Manager, Imperial College London
As the effects of climate change accelerate, risk managers in government and private enterprise are faced with increasing challenges as they seek to mitigate an uncertain future.
In May this year, the European Forest Fire Information Service reported that 2022 was the second-worst wildfire season on record, surpassed only by 2017. Given the current situation in Greece, this benchmark will be surpassed before long. And over four days in early June, the global average air temperature record was broken three times; previously, this had only happened twice over the preceding six years.
In other words, the pace of change is rapid and accelerating. And with vast human, ecological and financial consequences – last year’s wildfire season was estimated to have cost €2 billion – there is a huge imperative to model and understand the hazards that might result. But doing so with enough accuracy and efficiency is difficult.
Global climate models, for instance, can perform hundreds of billions or even trillions of calculations to model large-scale weather patterns at half-hour intervals over a hundred years – but can’t realistically be run at a high enough resolution to satisfy a risk manager seeking to understand the variation in storm surge probability along a particular coastline.
And with a run time of weeks or months depending on the hardware available, the process is difficult to iterate effectively. Iteration – running the same predictive model a number of times – is vital to the accuracy and reliability of its results.
It’s also challenging to keep track of unfolding events in real time. A huge range of resources are available to track wildfires as they unfold – from satellite arrays to social media. But cleaning, weighting and integrating noisy and heterogeneous data sets is a real challenge under such intense time pressure.
At Imperial, experts across a range of disciplines have found that AI tools can help them address these challenges: to improve long-term modelling for events like hurricanes and tropical cyclones, hedge risk in vulnerable investments like forestry, and create systems that can model natural hazards as they develop – and the effects of potential responses, too.
Machine learning offers unprecedented power to understand complex relationships between the enormous numbers of variables in current climate models. Natural language processing enables crowd-sourced information to direct satellite surveillance; and convolutional neural networks can process the resulting information with striking accuracy. Digital twins allow responders to simultaneously trial a range of measures against an unfolding emergency.
And as the frontiers of this work continue to develop, researchers continue to improve the explainability of AI-based predictions, as well as ensuring that they reflect real conditions grounded in physics as well as statistical probabilities…
Full report (3000 words) available on request